June 24, 2020
Letters from the Spark+AI Summit
COVID19 has seemingly pushed almost a decade of technology change in a short span of 2-3 months. If we are going to be a data driven society, then just having compute platforms is not enough, you need to onboard and retain sticky business workloads such as analytics, machine learning, devices, streaming etc. that will drive society.
Let me start by defining Business Analytics as the ability for organizations (of empowered individuals) to make reliable and dependable business decisions in order to drive business outcomes. This is not about report, it’s about business outcomes. It’s not about visualization, it’s about effective decision making. Let’s briefly, explore where we came from to see what the future may hold.
In times gone by, traditional business intelligence(BI) has been the place for businesses to go to ask questions of their messy and massive amounts of data. Traditional BI was painfully slow, for the needs of business. By the time a report was ready, business had moved on. There were more questions which required answers that meant additional development time for Traditional BI team before they can answer the new questions. The challenges lay in data wrangling, data engineering and ultimately creating a report specific schema using ETL that would allow a report to be built. This took weeks or months.
Fast forward to 2010, innovations in Visualizations from Tableau and other vendors started challenging the traditional BI industry by empowering business users to build analytics very quickly, in a self service manner. Business users started building visualizations that allowed them to ask questions of their data in a matter of hours or days instead of months.
While companies like Tableau (acquired by Salesforce $15.7 billion), Qlik (acquired by Thomas Bravo ~$3 billion), Looker (acquired by Google $2.6 billion) and others innovated the visualization and analytics layer, the struggle on the data side continued.
Challenges with data volume, velocity and variety were approached by many Big Data vendors by building data lakes. However the big data systems had many problems around data quality, performance and manageability.
Today, at Databricks share a lot of news about its vision for a Unified Analytics and Data platform at the Spark+AI Summit.
Databricks Delta Lake brings some key capabilities such as ACID transaction support, Schema validation, Indexing that are missing in traditional big data data lakes. This allows for ML, Analytics workloads to become meaningful in applications with more accurate, reliable, consistent and timely data driving accurate and reliable decisions in organizations
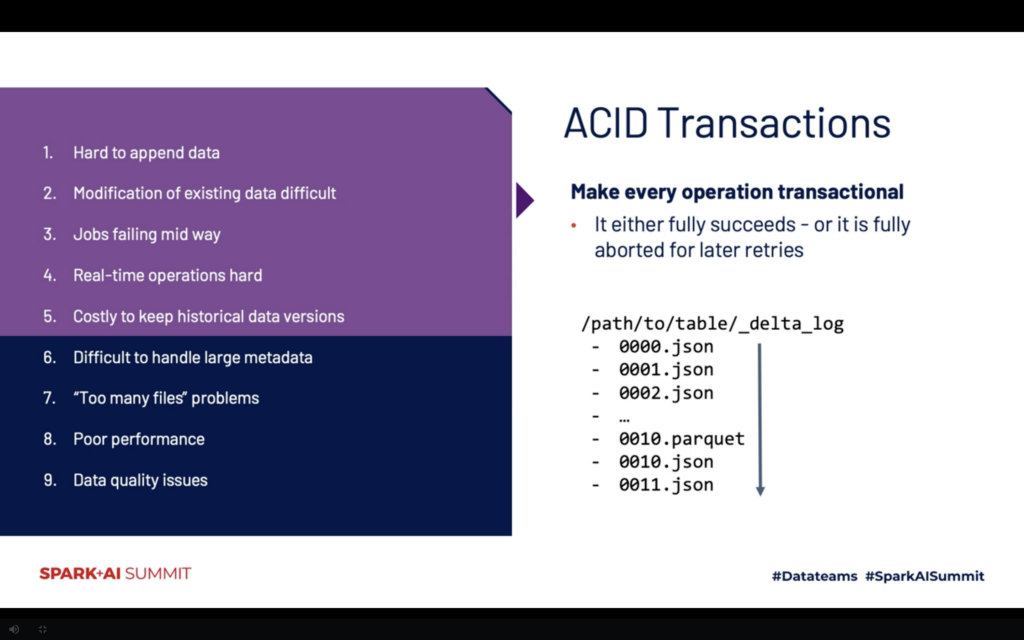
Apache Spark’s evolution over the last decade enables a lot of innovation that is driving data platforms to be managing data effectively for use for analytics and other business workloads.
Performance is one of those attributes that when it’s there, no one cares about it. However, when performance is missing, everyone is frustrated because things are so slow.
Apache Spark’s evolution over the last decade enables a lot of innovation that is driving data platforms to be managing data effectively for use for analytics and other business workloads.
Performance is one of those attributes that when it’s there, no one cares about it. However, when performance is missing, everyone is frustrated because things are so slow.
Delta Engine gives Delta lake a boost in performance by supporting vectorization using SIMD transaction set allowing better use of the CPU resources by driving parallelization in data and instruction set. This addresses the significant gains in performance in the storage and I/O space relative to the CPU clock speed that has become the bottleneck.
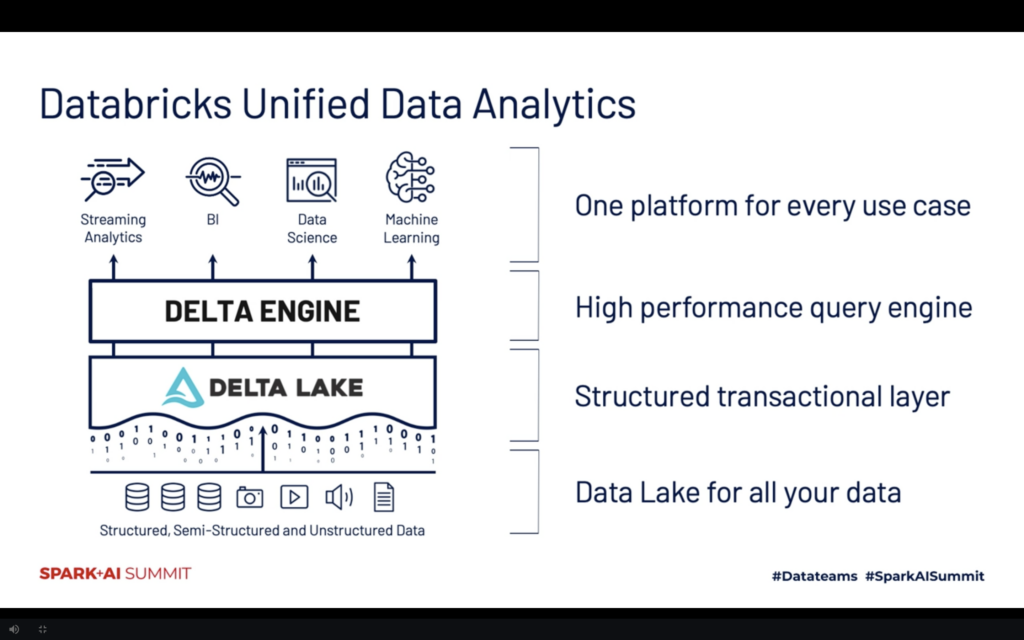
Databricks platform has also added a lot of connectors to allow smooth integration with business intelligence, machine learning and data science workloads.
Databricks announcement of Redash joining Databricks opens up more integrated and powerful native visualization technologies on the Databricks platform bringing it one step closer to it’s Unified Data and Analytics Platform vision
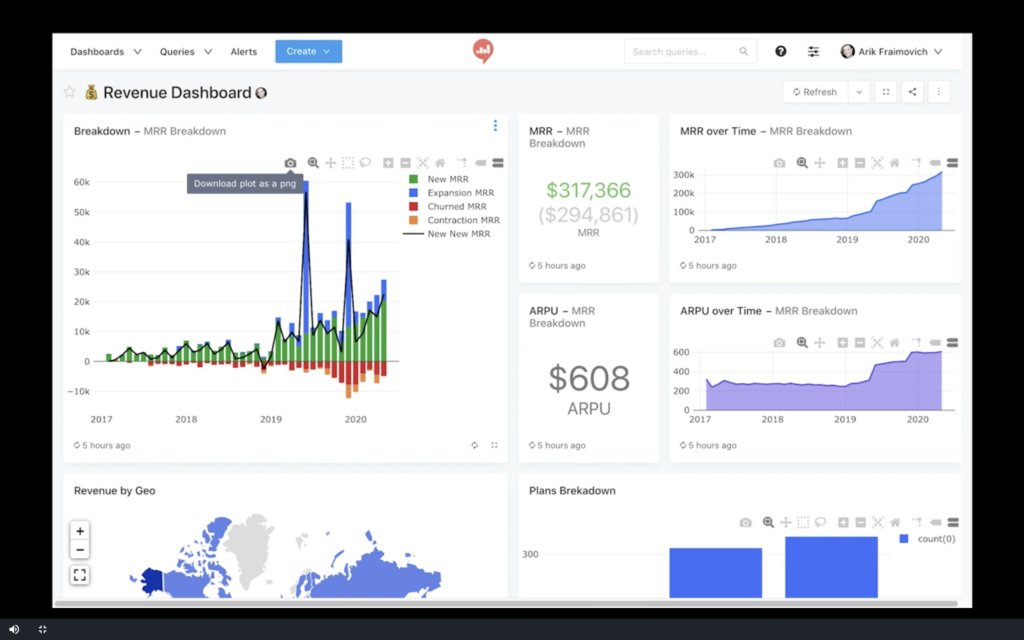
One cannot help but observe that over the years, as Analytics and Visualization platforms struggled with the problem of messy data, Data platforms saw the need for business workloads to keep the data platforms growing. While vendors like Tableau have moved into end user data preparation technologies with their Tableau Prep technology, one can see that Databricks is not only integrating with BI/ML workloads but embracing communities that provide easy to user interfaces on top of the data platform.
As Analytics companies invest in data technologies, and Data companies invest in visualization and analytics technologies, time will tell if these two markets are converging.
While the traditional big cloud vendors (AWS, Microsoft and Google) are clearly driving the IaaS/PaaS moves, emerging SaaS providers like Salesforce have acquired business workloads that have proven to be sticky over the years via acquisitions like Tableau. One thing is clear, Analytics without reliable data is meaningless, and data without business workloads like analytics is – a bunch of blinking lights on a server doing nothing.
Business Analytics As Service would allow for low cost subscription based models that enables all organizations (small, medium or large) to move to a reliable, dependable business analytics culture where data silo’s are broken and one platform is powering all the decision making analytics for all business workloads.
The next 3-5 years will prove to be interesting as the Business Analytics evolves to embrace the collapsing of the gap between Analytics and Data platforms.